Recombeelab's 2023 Research Publications

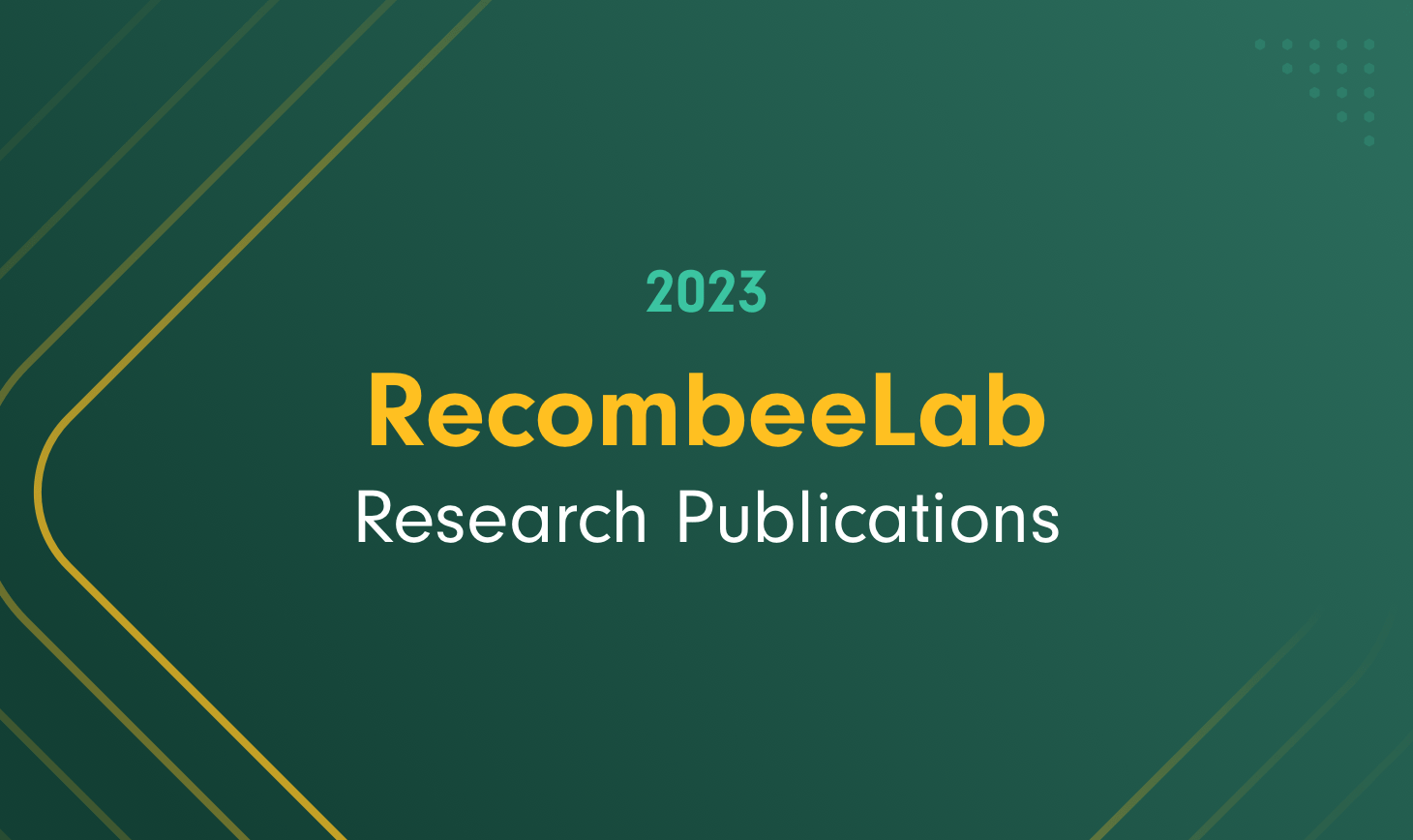
Recombeelab, a joint research laboratory of Recombee and the Faculty of Information Technology at the Czech Technical University in Prague, experienced a highly productive year in 2023, publishing a series of insightful and impactful papers in the field of recommendation systems.
Our research spans various types, ranging from (A) basic research, where we aim to understand phenomena without immediate practical application, to (B) fundamental research, where we develop methods applicable to general tasks, and (C) practical research, where we address specific problems (often tied to customer needs).
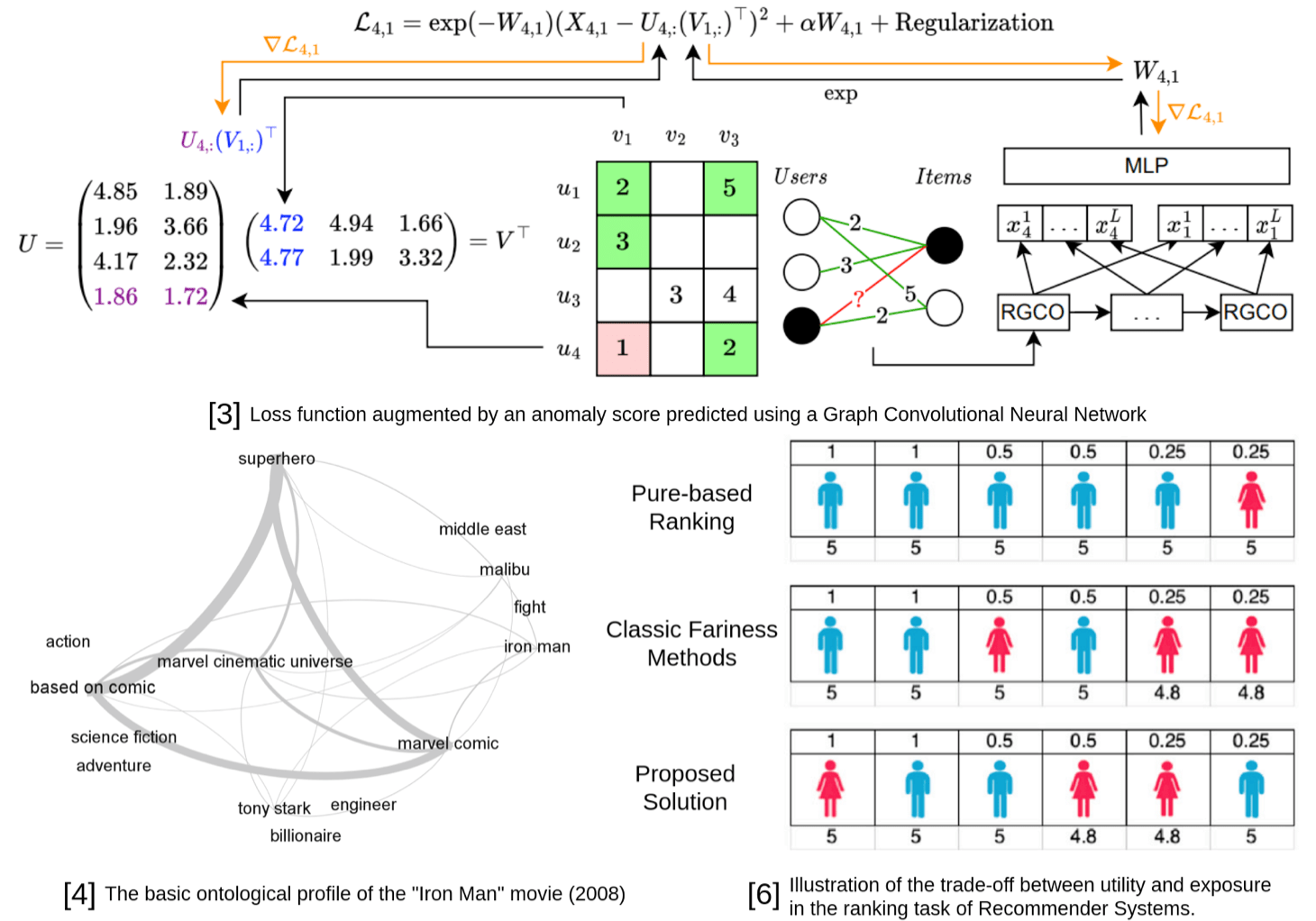
In the first category (A), for example, we made a significant contribution by introducing generalization bounds for inductive matrix completion in low-noise settings [1]. This contribution is important to the field as it establishes a theoretical framework for comprehending the performance of matrix completion algorithms under various noise levels. In simpler terms, the authors of these papers aim to understand how well the prediction performance of matrix completion (a vital method for recommender systems) behaves in different scenarios. Similar insights are presented in [2], where the focus is on understanding how the model behaves given uncertainties in the observation of user-item interactions. This involves scenarios where some users may be more reliable than others when rating items in the catalog.
In the realm of (B), fundamental research, our researchers have made valuable contributions in various aspects. For example, in [3], the authors propose a new factorization method that incorporates graph neural networks into the learning process. This algorithm proves to be more adept at handling incomplete data and is more robust to outliers compared to traditional matrix completion algorithms. Furthermore, [4] introduces a novel approach to addressing the cold-start problem in recommendation systems. This method utilizes ontologies and knowledge graphs to infer user preferences from incomplete data. Lastly, [5] presents a new algorithm designed to enhance the diversity and serendipity of recommendations in cold start environments. Employing an ontology-based approach, this algorithm identifies and recommends items likely to pique the interest of users.
Finally, in the domain of (C), practical research, Recombeelab also has made significant contributions. For example, in [6], the authors proposed a framework to minimize biases, including gender bias, ensuring a fair ranking that balances the visibility of items across all groups. For instance, traditional recommender systems often exhibited bias towards male candidates for high job positions due to biased data. However, this method focuses on providing exposure guarantees for all involved groups, addressing biases and aligning with the principles of responsible AI in today's context. Moving on to [7], the authors aimed to enhance our understanding of the real-world performance of recommender systems by introducing a new metric for evaluation. This metric is time-dependent and free from popularity bias, making it more accurate for real-life scenarios. Additionally, [8] extends a shallow autoencoder (also developed by our group) algorithm for collaborative filtering. This scalable and easily explainable algorithm proves to be a practical choice for real-world recommender systems. Moreover, [9] introduces a new algorithm designed to improve the diversity and serendipity of recommendations in cold start environments. This algorithm employs an ontology-based approach to identify and recommend items likely to be of interest to users.
Overall Impact
These publications represent a significant contribution to the field of recommendation systems. They provide new theoretical insights, propose novel algorithms, and address important theoretical and practical problems. Recombeelab is one of the leading research laboratories in this field, and our work has the potential to significantly improve the performance and usability of recommender systems.
Regarding the venue of publication, RecombeeLab has submitted to high-impact journals such as the IEEE Transactions on Neural Networks and Learning Systems and Expert Systems with Applications. As for conferences, in the year 2023, we have published papers in premier recommender systems conferences such as ACM RecSys and the prestigious AAAI Conference on Artificial Intelligence (considered one of the top-5 best conferences in the AI field). These journals and conferences are widely recognized by the research community for their impactful contributions, and their high rejection rates indicate the rigorous selection process ensuring only significant research is accepted. One highlight is the Best Student Paper award received by Petr Kasalický in the Recommender Systems Evaluation Workshop held at the prestigious Conference on Knowledge Discovery and Data Mining (KDD). Kasalický, an industrial PhD student in RecombeeLab, is actively involved in important projects crucial to Recombee.
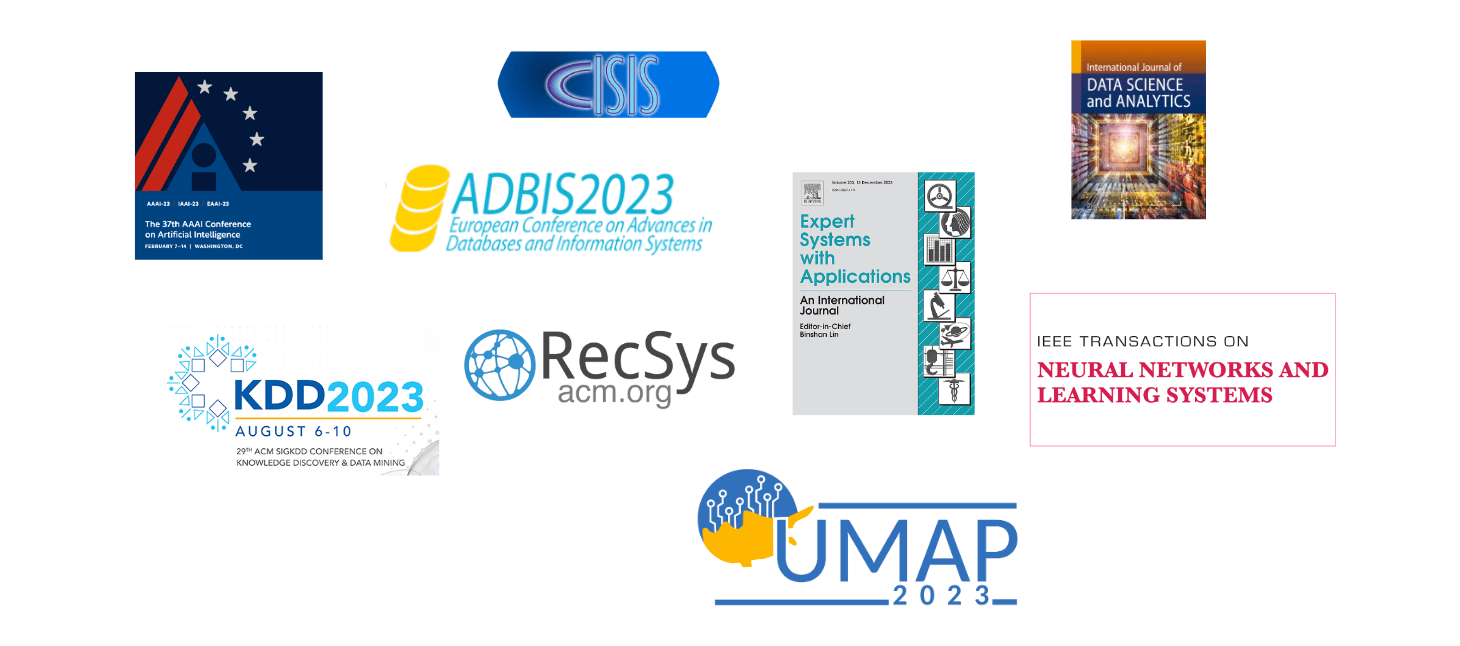
We strongly believe in the necessity of building an international, inclusive, accessible, multicultural, and prejudice-free environment for research. In 2023, our international research collaborations were co-authored by individuals from seven different nationalities and affiliated with eight institutions located in four countries. We remain committed to exploring new collaborations and making our lab accessible to researchers from diverse backgrounds.
If you are interested in learning more about our research, please feel free to contact us.
References
[1] Ledent, Antoine, Rodrigo Alves, Yunwen Lei, Yann Guermeur, and Marius Kloft. "Generalization bounds for inductive matrix completion in low-noise settings." In Proceedings of the AAAI Conference on Artificial Intelligence, vol. 37, no. 7, pp. 8447-8455. 2023.
[2] Alves, Rodrigo, Antoine Ledent, and Marius Kloft. "Uncertainty-Adjusted Recommendation via Matrix Factorization With Weighted Losses." IEEE Transactions on Neural Networks and Learning Systems (2023).
[3] Kasalicky, Petr, Antoine Ledent, and Rodrigo Alves. "Uncertainty-adjusted Inductive Matrix Completion with Graph Neural Networks." In Proceedings of the 17th ACM Conference on Recommender Systems, pp. 1169-1174. 2023.
[4] Kuznetsov, Stanislav, and Pavel Kordík. "Overcoming the cold-start problem in recommendation systems with ontologies and knowledge graphs." In European Conference on Advances in Databases and Information Systems, pp. 591-603. Cham: Springer Nature Switzerland, 2023.
[5] Kuznetsov, Stanislav, and Pavel Kordík. "Improving recommendation diversity and serendipity with an Ontology-based algorithm for cold start environments." International Journal of Data Science and Analytics (2023): 1-13.
[6] Lopes, Ramon, Rodrigo Alves, Antoine Ledent, Rodrygo LT Santos, and Marius Kloft. "Recommendations with minimum exposure guarantees: A post-processing framework." Expert Systems with Applications 236 (2024): 121164.
[7] Kasalický, Petr, Rodrigo Alves, and Pavel Kordík. "Bridging Offline-Online Evaluation with a Time-dependent and Popularity Bias-free Offline Metric for Recommenders." arXiv preprint arXiv:2308.06885 (2023).
[8] Vančura, Vojtěch. "Scalable and Explainable Linear Shallow Autoencoders for Collaborative Filtering from Industrial Perspective." In Proceedings of the 31st ACM Conference on User Modeling, Adaptation and Personalization, pp. 290-295. 2023.
[9] Žid, Čeněk, Pavel Kordík, and Stanislav Kuznetsov. "Personalised Recommendations and Profile Based Re-ranking Improve Distribution of Student Opportunities." In Computational Intelligence in Security for Information Systems Conference, pp. 217-227. Cham: Springer Nature Switzerland, 2023.
Next Articles
Is This Comment Useful? Enhancing Personalized Recommendations by Considering User Rating Uncertainty
Picture this: you're on the hunt for the perfect new smartphone, browsing through your favourite online electronics store. The online store’s recommendation engine pops up with what it thinks could be your possible next gadget love...

AI News and Outlook for 2024
We look at the most interesting research directions and assess the state of knowledge in key areas of AI. We'll also estimate future developments in 2024 so you know what to prepare for.

AI Assistants Know Your Preferences, Even Better Than You Do
Recommender systems and ethical controversies
